Artificial intelligence (AI) has become one of the most popular modern technologies in the world today because of the potential it holds to make everyday tasks easier and error-free. Almost all tech giants are adopting AI to carry out various tasks. Another interesting aspect that these companies are investing in is leveraging AI solutions to reduce their CO2 footprint on Earth, thereby, making their services more sustainable.
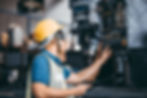
Using AI to Reduce Resource and Energy Consumption
As humans progress in life, they develop systems to make tasks more convenient. But they fail to factor in the effective increase in the level of CO2 in the atmosphere. This imbalance paved the way for climate change, a phenomenon that will have drastic effects soon if humans don't take steps to help it.
AI can be widely used to make the environment cleaner and safer for its inhabitants. Here are a few ways different industries are using AI to reduce carbon footprint:
Agriculture Sector
AI can be used in the agricultural sector to monitor and manage environmental conditions and reduce water and fertilizer consumption while improving crop yields.
Weather Prediction Systems
AI-powered prediction systems and intelligent grid systems are used to control the supply and demand of renewable energy resources. More accurate weather predictions by AI models increase efficiency by reducing costs and avoiding the production of unnecessary CO2.
Transportation Industry
AI monitors congestion on roads, which improves transportation systems and also provides support to autonomous driving systems.
Water Supply
By monitoring water usage through AI models, water wastage can be reduced while cutting down costs.
AI can aid in designing more efficient systems that consume significantly less energy and produce less waste.
Electric Power Industry
AI reduces energy consumption in buildings by keeping track of the number of people and the amount of non-renewable energy at disposal.
Solar Panels Manufacturing
AI can be used to find improved material for use in solar panels to convert heat to useful energy. Not just that, but CO2 absorbent materials can also be discovered through AI.
How Companies Leverage Industrial AI to bring Sustainability
The list of use cases AI has, to produce a more environmentally friendly way, goes on for miles. Various companies around the globe are going green, and many are making use of AI to do so. Some examples around us include:
Waze and Google Maps made smart transport possible by providing real-time data on traffic flow and congestion, ensuring that vehicles are in transit for a shorter amount of time, releasing fewer emissions.
Blue River Technology combined satellite images and AI to pinpoint the appearance of invasive species in any habitat, along with other changes in biodiversity.
Google developed DeepMind AI, which lessened the energy consumed in cooling their power-intensive data centres by 40%.
IBM increased the accuracy of its weather predictions by utilising AI models. This aids the effective management of plants and makes renewable energy production more efficient, reducing harmful CO2 emissions substantially.
The non-profitable think tank, Carbon Tracker, has an AI model that utilizes satellite images to trace carbon emissions of various coal plants. It helps drive investments more towards eco-friendly ventures by providing awareness.
Use Cases Of Predictive Maintenance, Predictive Quality and Visual Inspection Reducing CO2 Footprint
This section will navigate what predictive maintenance, predictive quality, and visual inspection are. We also jump into how here, at craftworks, these solutions are developed to contribute to eco-friendliness in the industry.
Predictive Maintenance
Predictive maintenance involves constant monitoring of any system in normal working conditions to predict any future failure. When system failure is anticipated by testing a set of predefined conditions, it is prevented by ensuring that timely maintenance is done.
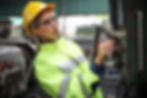
craftworks was approached by a sizable district heating network. It comprised thousands of converter stations responsible for cooling the water that is later delivered to customers. They complained of frequent and sudden system downtimes, which caused a decline in their productivity and efficiency.
The professionals at craftworks produced a machine learning algorithm that utilized past data to predict system downtimes seven days before it actually happened. This made it possible for the company to schedule maintenance on time and avoid downtime in the first place.
Not only does the algorithm predict downtime, but it also finds connections between downtimes and sets of particular past data. This information educated the company’s top management about the causes of the downtimes and how they can improve their systems to reduce the possibilities of system failure altogether.
This algorithm initially forms a recommendation system and can be further scaled to set parameters automatically to boost system efficiency.
Predictive Quality
Predictive quality applies statistics and machine learning algorithms to the test data obtained from various sources to deduce useful information, such as trends, patterns, and future outcomes.
This practice aids in predicting failures, bottlenecks, productivity reduction, errors, etc. in systems. Such information supports professionals during decision-making while dealing with various projects in the testing phase to improve their overall quality.
craftworks had the opportunity to collaborate with an international brick-manufacturing company. The production line consisted of grinding and milling the material for the brick, after which, it was shaped and dried down to take on a sturdy form. The company presented interest in decreasing the scrap output rate in one of its production plants.
After an assessment, the professionals at craftworks identified that quality control at the company was only done at the end, making it complex to determine the root cause of the scrap output. There was no way of knowing what could trigger the manufacturing of a faulty brick at any point. The unpredictability gave rise to inefficiency throughout the process.
As a solution, the quality data from the machine was paired with the data coming from the sensors during different production steps. A pattern was formed that ultimately enlightened the employees working at the company about when, where, and why the fault occurred.
craftworks’ quality control AI predicted potential problems during the production line. The use of real-time data gave valuable insights as to what would be the optimal settings for the production of high-quality bricks. Consequently, the scrap output rate was reduced significantly.
Visual Inspection
Traditional visual inspection involves professionals assessing objects and detecting faults. Of course, the method is not 100% foolproof as human error is inevitable and their speed and expertise are limited. The AI-based visual inspection overcomes the pitfalls of the traditional one.

AI-based visual inspection consists of feeding images of an object to a machine learning model. Consequently, the model analyzes the image and detects any anomalies present. This method is used for speedy and reliable quality assurance.
craftworks was working on a case of an international automotive supplier, which manufactured various vehicle components. There’s no room for error in the manufacturing of these car components, as they must comply with high-quality standards. The goal was to develop a highly efficient automated visual inspection system for analyzing the metal components produced at the end of the production line.
The existing visual inspection system of the automotive company worked well enough, but required intervention by humans due to its high pseudo scrap rate. So the potential efficiency of the system remained untapped.
craftworks' AI-based inspection system utilises industrial camera images for the detection of defects in automotive parts. This system uses a semi-supervised approach, i.e., most of the data used to train the model are unlabeled.
The system easily differentiates between defective and working parts. It can further learn the different types of defects and improve their accuracy while classifying defecting and working components. The system produces a significantly lower pseudo scrap rate, making efficient automated quality control possible. Now, the company no longer requires their employees' time for quality control!
Parting Thoughts
Today, human beings cannot survive without the technological advances made in the world in recent years. Though, it is wise to admit that we hold the responsibility for the outcomes of these advances.
Artificial intelligence has a wide scope, which can be exploited to the fullest to make the world technologically modern and environmentally friendly. Take a look around you, and you’ll see hundreds of companies contributing to making their services and products more sustainable in the long run.
There’s so much more that can be done, especially in the industrial sector, to lower the carbon footprint in the world. We all need to work together to bring sustainability and take steps to make our Earth environmentally friendly. For more solutions that have an impact on the sustainability of your company, visit our success stories page. Here you can find concrete examples of solutions we have developed for our customers.